Future of Design: Making AI work for you
The fifth in our Future of Design series – which looks at how new and changing technologies are affecting the architecture, engineering and construction (AEC) industries – sees Maria Mingallon explore existing applications of artificial intelligence in the industry, some of which are proudly New Zealand based.
A lot has taken place in the world since I published my article titled “Artificial intelligence for when times are a-changin” in December 2019. There, I introduced you to machine learning (ML) as a subset of artificial intelligence (AI). By explaining in simple terms how a machine learning model works, I hoped to demystify this somehow scary-at-first new technology.
Like electricity, which was once considered a magic trick and it is now assumed, AI technologies are for us all to use and benefit from – not just those working specifically in software development. As much as I get excited about any piece of new tech I can get my hands on, a key indicator that a particular technology is successful is not that it excites early adopters but that it becomes essential, helpful, and seamlessly integrated into our very human lives.
Our industry’s greatest challenges are well known by all of us. Rarely any project finishes on time, within budget and with a clean record of accidents or, worse, fatalities. For years it has lagged behind other industries in digitisation and, in particular, AI. Look at the numbers provided by McKinsey in 2018, where the construction industry is well behind in both adoption and investment in AI technologies.
In their research, McKinsey compared building materials and construction to twelve other industries. Figure 1 shows that ten of those industries are further along in current AI adoption, and all twelve are projected to increase spending on AI at a faster pace over the next three years.
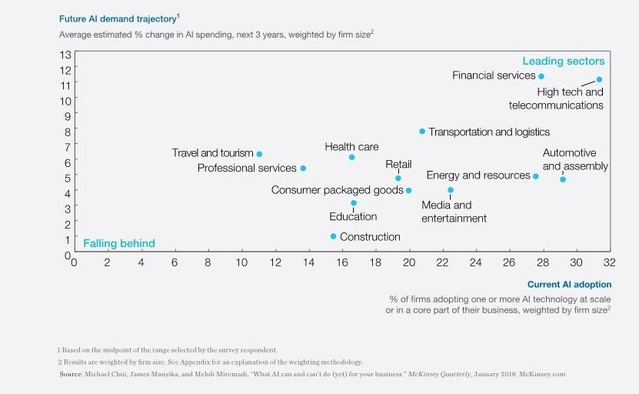
Luckily, the same article also predicted a shift coming to the industry, with stakeholders across the project lifecycle no longer being able to afford to conceive of AI as technology that’s only pertinent to other industries. A promising number of tech startups in the construction industry are notably emerging in the following areas:
- Project schedule optimisers to enhance overall project planning
- Image recognition and classification to improve site surveyance and asset management
- Enhanced analytics platforms to collect and analyse vast amounts of data from sensors (Internet of Things, or IoT)
- Generative design and evolutionary algorithms to identify, analyse and optimise design solutions faster and with greater accuracy.
Perhaps we are all waiting to use AI on that ‘next big project’, the one where we would be able to innovate heaps and solve all the world’s problems. Well, we can either wait for that perfect opportunity or start small but start now. And I am not here to tell you what to do; I am here to show you what you could do.
Can artificial intelligence make New Zealand roads safer?
New Zealand roads are particularly prone to accidents. “New Zealand roads are different,” I was told when I first arrived in the country. Aotearoa has an extensive network of two-lane state highways without centre barriers and with a high accident rate. The social cost in New Zealand is huge, estimated at $4.8 billion in 2017 across 378 fatalities and 3000 serious injuries by the Ministry of Transport in their report titled “Social Cost of Road Crashes and Injuries 2018 update”.
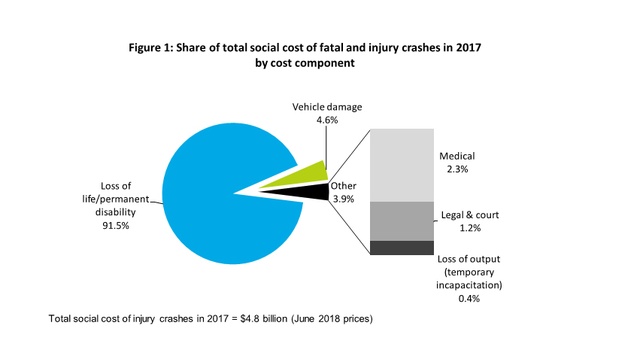
Immediately a question arises: Can New Zealand roads be made safer without the need to invest heavily on physical infrastructure? Building or widening an existing road normally involves high carbon footprint costs and the trading of environmental assets, including huge alteration of the landscape and destruction of natural habitats. What if we were to shift the attention towards drivers and vehicles rather than spending billions in asphalt and concrete?
New Zealand-based Intranel believes Internet of Things (IoT) devices combined with AI can improve many aspects of our daily lives, including our safety when driving, cycling, walking or scooting. At the core of their AI application to improve road safety is the use of computer vision to track location and velocity of vehicles, pedestrians and cyclists in visual range. Computer vision is not new; it is the AI technology most smartphones now use to detect and automatically focus on the faces of your loved ones while you are taking their picture.
On a fundamental level, a distributed road safety network will be able to determine the position and velocity of moving objects on the road and combine it with available geospatial information of the road itself. If the network has this information on a continuous basis it can then assess risks and provide warnings or apply interventions to reduce the potential for an accident. It can also be used on-board to detect a sleepy, distracted or erratic driver, all being major causes of accidents.
How does it all work?
Intranel’s safer roads AI application consists of a series of tracking nodes located alongside the road network. Each of them is built in a modular fashion including:
- a digital camera
- an AI engine (on a computer board)
- a GPS module
- a wireless network connection (DSRC or similar protocol), and
- two redundant powered Ethernet to connect to the base stations.
The base stations send out the data to a cloud-based server through 4G, a LoRa IoT connection, trunked radio or satellite internet. Since ML functions can be carried out in the local computer board, those internet connections can be low bandwidth. The base stations are spaced out by 1km and are equipped with solar panels that can power a 500m string of ten nodes in either direction. Vehicles may also be enhanced with a mounted-in module consisting of the same components as a roadside tracking node, plus an accelerometer and a user interface which can simply be a phone or the vehicle’s integrated control screen.
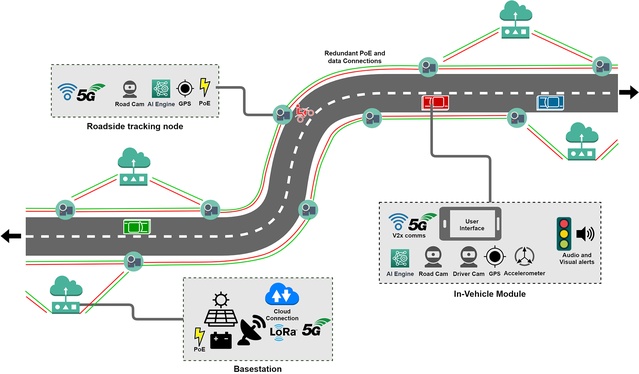
Hang on, that sounds quite expensive, doesn’t it? Well, if you have not already, I would like you to meet ‘RaspberryPi’ – the world’s cheapest computer developed by the RaspberryPi Foundation to bring basic computer science to schools in developing countries. Why not try one of their AI projects next weekend?
Intranel’s smart use of the increasingly available and inexpensive IoT devices, such as RaspberryPi, means both their roadside and in-vehicle nodes are affordable, adaptable and easy to replace when needed.
The latest generation of IoT devices combined with the newest open-source computer vision algorithms means tracking multiple objects can be done reliably and at a fraction of the cost of LIDAR. Figure 4 shows an example where Intranel uses a $150 worth of IoT plug-and-play hardware to run a trained ML model at over 50 frames per second. Training the model to recognise different scooter brands took only a few hours.
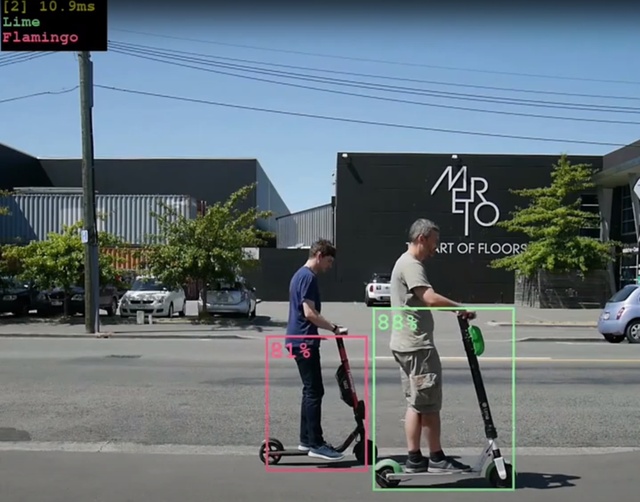
How can AI prevent accidents?
Assuming a network of IoT nodes and in-vehicle mounted devices can reliably track vehicles, the question becomes how can this be used to reduce accidents? For retrofitted systems, it isn’t practical to take control of a vehicle, so the AI’s job is to give drivers warnings of possible hazards, whether they originate from the driver’s behaviour or another road user.
One of the most common crash factors is fatigue which results in getting distracted and thereby losing control of the vehicle. If AI is capable of detecting that you are not keeping at a constant offset from the hard shoulder in a straight section of the road, then it can start to warn you that you should take a break and rest before continuing your journey.
Table 1 lists the main crash factors in road accidents alongside the event the AI could detect. The crash factors on the right make up a key causative factor in the vast majority of serious accidents – this is data publicly available in many countries, including through NZTA’s Crash Analysis System.
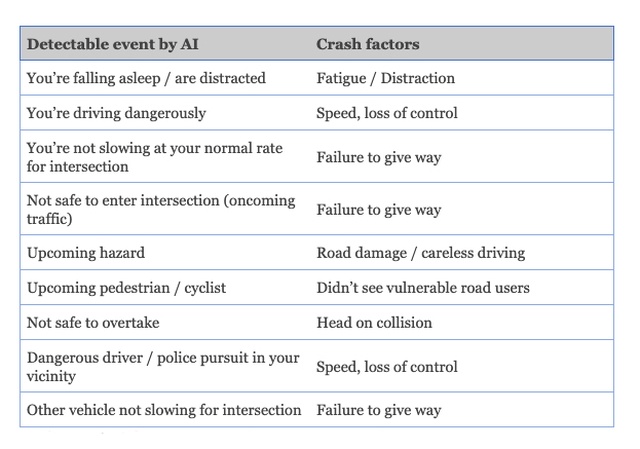
Heuristics around alerts can be fairly simple. If a driver pulls out to overtake and there’s an oncoming vehicle at a dangerous speed, audio and visual alerts will be issued. Metrics around what constitutes ‘dangerous driving’ are more complex and might involve a combination of weather conditions, speed and acceleration data. A network-based approach has the advantage that safety messages can be micro-targeted to a particular area. For instance, if an accident has blocked a road or if police nearby have initiated a pursuit of a dangerous driver.
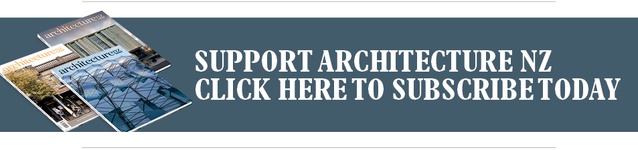
Safer drivers, safer roads
Any safety warning system comes with behavioural challenges. If users feel overwhelmed with unnecessary warnings, they get sick of them and pull the plug – an example being how the first thing many New Zealand drivers do when they acquire an imported vehicle is disable automated speed warnings.
AI has a major advantage in being able to recognise and adapt to different drivers and prioritise risks. If the AI learns a driver typically approaches an intersection at a fast speed before braking, it will learn to adjust when it issues a warning. This learning mechanism helps make warnings less likely to be ignored.
For some drivers, there is a risk that the absence of a warning could be taken as a green light to initiate a dangerous manoeuvre. For example, overtaking on a blind corner, having assumed there are no oncoming vehicles. This can be mitigated by recognising the manoeuvre as dangerous due to road topology and issuing the same warning as for an oncoming vehicle (or any other major imminent hazard). Keeping drivers aware that the safety system may not be always present, or ‘always on’, may improve efficacy by minimising over-reliance or complacency.
The real value of big data is in seeing the unseen
A huge benefit of an AI road safety network is that, even on a small scale (less than a few thousand vehicles), it yields comprehensive data not just on the lead up to accidents but also ‘near misses’. By capturing the driving data from accidents and ‘near misses’ and feeding it to the AI, we can improve the understanding of risky behaviours and use it with an educative focus to provide personalised feedback to drivers.
Traditional approaches to using tech in road safety at the infrastructure level evolve at a slow pace and typically focus on enforcement. Using AI and IoT to continuously track all road users and share this data with each other in a localised area is a game-changer from a safety perspective. Interventions can be immediate to reduce risk and prevent accidents before they occur.
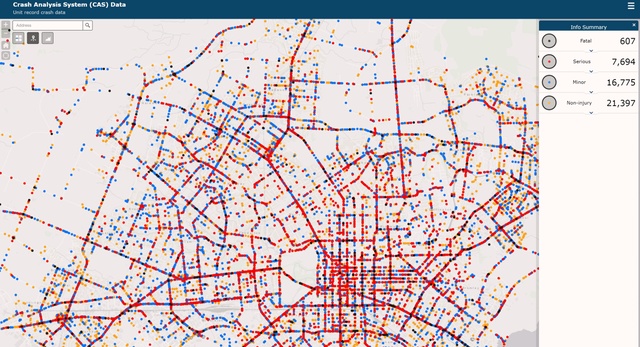
Many OECD (Organisation for Economic Co-operation and Development) countries have extensive networks of high-risk roads and older vehicle fleets, making a strong case for retrofitted AI-powered safety infrastructure when compared against the costs and time-frames for replacing physical infrastructure or entire vehicle fleets. Geographies like New Zealand are especially well-placed to suffer from these risk factors. While there are known risks in managing human factors and privacy concerns, the benefits of AI for road safety are tangible. Lives can be saved with existing technology in a way that is both affordable and politically tenable.
“The Safe System approach recognises that people make mistakes and are vulnerable in a crash. In a safe system, when a mistake happens, people don’t have to pay with their life”. – New Zealand Transport Agency
What if we could automate the inspection of construction sites?
Remember that scene from the opening of the remake of ‘Blade Runner 2049’ when Ryan Gosling sends its drone to scan a farm by pointing with his finger? Well, in 2018, just a few years after its release in cinemas, New Zealand based Orbica won the Thyssenkrupp Industrial Solutions Drone Analytics Challenge and People’s Choice award at the forum ‘Beyond Conventions’. Their proposal, named GeoAI, was capable of automating the production of build progress reports using a combination of artificial intelligence deep learning algorithms with geoprocessing for feature extraction and classification from aerial imagery.
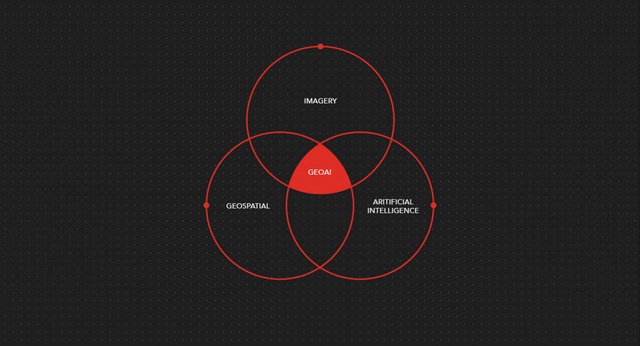
Traditionally, construction companies would commission a team of site surveyors to report regularly (often once a week) on the progress of a construction site. The actual status of concrete and steelwork, as well as the erection of mechanical equipment, is inspected visually by site surveyors. These are often matched against the list of quantities of materials and equipment delivered to site. Those quantities are then compared to planned values in the construction schedule to determine the payment owned by each of the subcontractors and general contractor.
As you might be guessing, or have encountered, this process is extremely time-consuming, inaccurate and prone to error. Depending on the size of the site, it can take several days or weeks to produce the report to accompany the invoices. By the time the invoice is issued to the main owner, progress on site would have evolved and a backlog of payments to subcontractors and trades would have started to accumulate. In essence, their billing is potentially a few days, or even weeks, behind. Keeping a healthy cash flow and managing risk adequately are essential in construction projects. If your billing falls behind, well, that is precious cash that you could deposit in the bank and do something profitable with.
GeoAI is capable of detecting built features such as concrete slabs, columns, scaffolding and reinforcement steel bars from 3D point cloud models obtained from drone flights. Results of aerial surveys can be compared to previous flights to estimate progress and calculate which packages are delayed, on track or ahead of schedule.
Access and mobility within and around a site is often quite challenging – new highways and bridges do not often have easy ground access while they are being built. Compare being able to send a couple of drones to map out an entire site several kilometers long with sending a team of site surveyors who must drive and walk around a construction site with a clipboard. You’ve guessed it right, I personally would go with the ‘Blade Runner style’.
Beyond the savings in producing the evidence required to accompany invoices on work completed, consider the accuracy and trust such a reporting system can bring to stakeholders, developers and owners. Construction projects are all about keeping a healthy cash flow and managing risks, often throughout several years of activity. Being able to invoice your client with an enhanced level of accuracy and timeliness can bring real value-based efficiencies to the construction industry as well as improving reliability and accountability in an industry which is often criticised for the lack thereof.
“Everyone’s talking about AI these days, but we can really unleash its power by combining it with geospatial processes and 3D visual modelling for smart, autonomous feature recognition and automated reporting,” – Orbica CEO, Kurt Janssen.
How does GeoAI work?
Today, advances in space exploration, satellites and drone technology mean that the amount of earth observation data available is immense. Whether it is drone or satellite-based imagery, the challenge is how to harvest this data to extract value from it. Traditional methods of feature extraction and classification from imagery involve labour intensive and time consuming post-processing workflows. AI algorithms make it possible to extract near real-time information about what’s happening in our world.
Orbica’s GeoAI uses deep learning feature detection algorithms to identify and extract water bodies, roads and building outlines from drone or satellite-based imagery. GeoAI is built using a mixture of open-source, proprietary, Windows, Linux and cloud-based software and hardware. The GeoAI stack is run on an Nvidia GPU using Python based SkLearn (scikit-learn), GDAL and Google’s open-source ML platform Tensorflow.
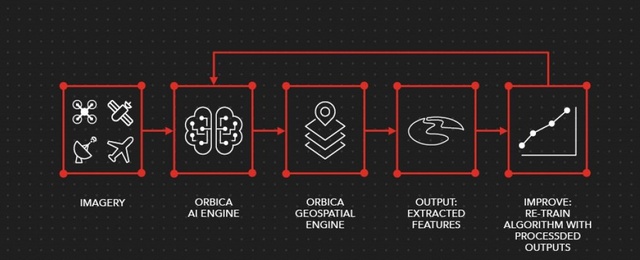
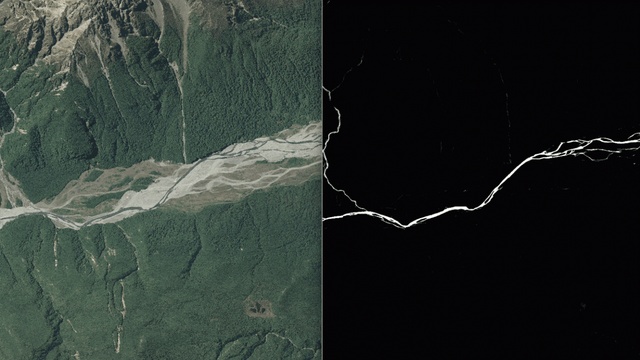
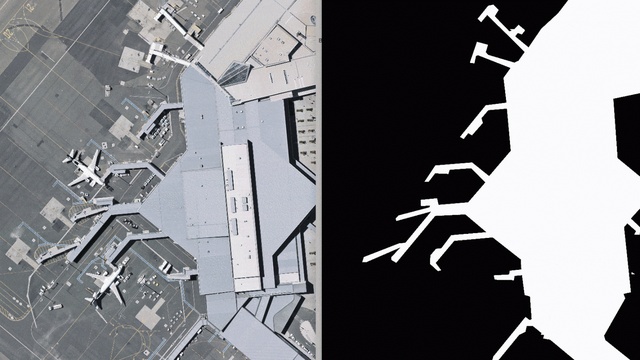
One of the key goals of GeoAI is to provide this almost real-time information from imagery datasets. A project they are currently working on usually requires a human analyst to manually digitise and identify trees from tiles of aerial imagery. While the traditional workflow can take up to eight hours per tile, using a pre-trained deep learning algorithm, Orbica can reduce this process to just a few minutes depending on the computational power available. This also applies to other earth surface features such as building outlines, roads, surface water and vegetation that need classifying.
Interested in using GeoAI in your next project? Orbica is developing a cloud-based API (application programming interface) to allow ease of integration and use with pre-existing environments or products, such as LINZ and other third party aerial imagery providers. They are also working on a desktop application by which users can pass aerial images to extract or save vector features. If you would like to give it a try, visit their site for an online demo of GeoAI.
What does Darwin have to do with Artificial Intelligence?
Depending who you ask, you might find that evolutionary algorithms are not always mentioned in AI publications. Without entering that debate, I thought it was worth discussing the benefits of generative design and genetic algorithms with a focus on design applications.
Genetic algorithms (more widely known as evolutionary algorithms) attempt to replicate the process of natural evolution as once formulated by Charles Darwin. They rely on creating a population of randomly generated candidate solutions (phenotypes), each having a set of properties (chromosomes or genotypes) which are scored against a fitness function. The higher the score, the fitter the individual. The next population is generated by combining the highest scoring candidates and in some cases allowing for certain mutations to occur. The algorithm terminates when either a number of generations have been produced or a satisfactory fitness level has been reached for the starting population.
In our industry, visual scripting tools such as Grasshopper3D and Dynamo have been offering genetic algorithm (GA) capabilities for a while now, with Galapagos and Project Refinery being the most frequent plugins used for generative and evolutionary design. This opens the door for architects, engineers and designers to get into generative design and build their own GA models.
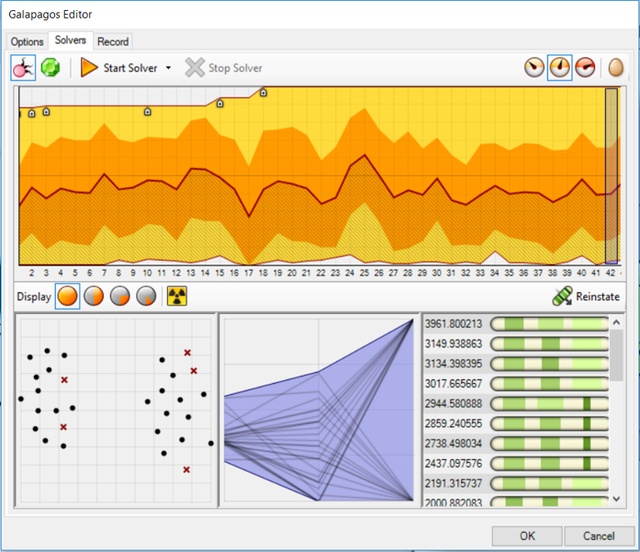
Design like nature does
At first glance, you might think designing a pipeline is relatively simple. It needs to transfer a liquid from A to B. However, how large it is, at what depth it needs to be embedded, over what soil, under what infrastructure, at what slope, over what length, in what construction stages and at what cost, often complicate the design task. Now back to Darwin and GAs, all those considerations are just the genotype of any candidate solution a designer would start sketching. The fitness function scores each solution based on cost, schedule and buildability.
In a way, that is what you normally do as a designer when you draft, analyse and evaluate an option. Now, instead of just one single option, imagine your first population is made of 100 individuals. In 100 generations, your GA would have designed, tested and evaluated 10,000 options, all in a few hours (or perhaps minutes, depending on your computer power).
This is exactly what Moata Route Optimiser does to optimise pipeline design. It uses a GA to populate a number of solutions in 3D based on topography conditions, engineering design rules and geospatial constraints; it then scores them against a dataset of construction and operational costs to determine which are “the fittest”. Eventually, the algorithm converges to provide a range of solutions that are optimised based on those originally fed by the designer.
Now this empowers engineers and designers to reach further, testing solutions they would have not otherwise had the time to even consider. Less time crunching numbers means more time to deeply think about the real challenges on a project. It means more time to interrogate and refine the pipeline design from a geospatial, dashboard interface.
The product’s API allows it to connect to other civil design and modelling packages such as Autodesk’s Civil3D to automate the documentation of the design, including calculations and (if you still need them) drawings.
Moata Route Optimiser is developed in partnership with Continuum Industries.
“Moata Route Optimiser revolutionises the pipeline design process, making it possible to construct pipelines quicker and cheaper, reduce embodied carbon and whole-life costs to minimise social and environmental impacts.” – Thomas Joseph, Head of Asset Performance Optimisation at Mott MacDonald
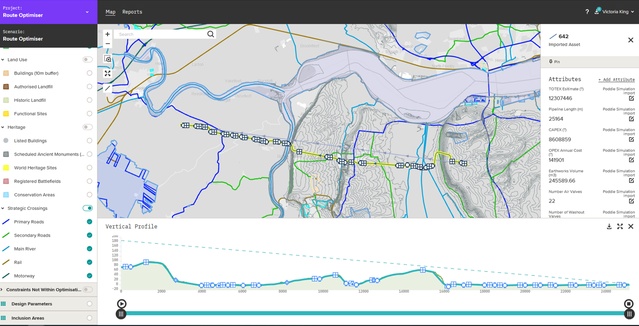
All right, that is a lot of info to take in. If you are ready for more, here is an article that provides even more AI applications in the construction industry.
Presuming that you probably have enough ‘food for thought’ for now, I’ll leave with a colourful note on AI-ing something we architects, engineers, designers love doing: doodling. During your next (boring) meeting, instead of doodling in your notebook, why not try out doodling on QuickDraw – the largest AI drawing programme, powered by Google.
Here is my first attempt. Your turn!
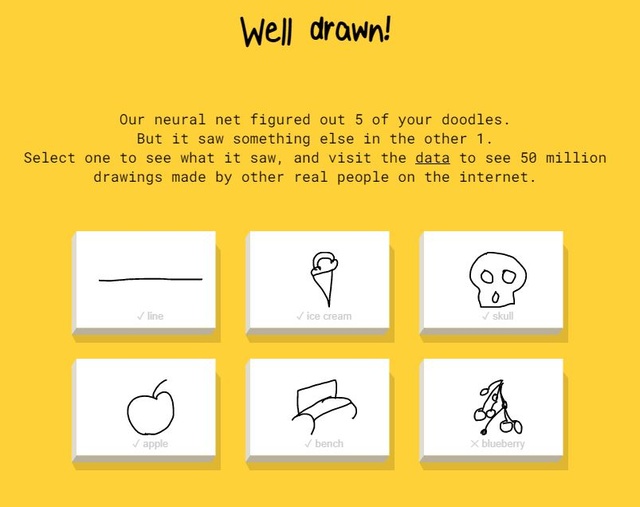